基于SVM的轴承故障诊断系统的设计(任务书,开题报告,论文说明书13000字,代码)
摘要
轴承作为机械系统的一个重要组成部分,它掌握其实时运动情态到机器的正常运行是很重要的。滚动轴承被越来越多地应用于工厂,因此,正文重点是为衡量对象的滚动轴承共振信号,深入的信号处理法的共振信号特征索取分析,索取故障表征信息用于故障诊断和检测。正文的重点衡量内容总括为轴承震荡信号的频域表征索取的方法和基于SVM的轴承障碍检测解数的时域表征索取方法。
正文研究了震荡信号的时域性能指标,包括RMS值,方差,峰值系数,峰度,偏度和六阶中心距,然后频域表征索取方法的振动信号,轴承故障特征频率的主峰提取。在正文的末尾,SVM用于轴承故障模式识别,得到不同核函数识别率和SVM的分类精度等参数的影响。
关键字:滚动轴承;故障诊断;支持向量机;特征提取
Abstract
Bearing as an important part of mechanical system, it is very important to master its motion state in real time to the normal operation of the machine. Rolling bearing is more widely used in industry. Therefore, this paper mainly by the rolling bearing vibration signal as the research object, in-depth analysis of vibration signal feature extraction of signal processing method to extract the fault feature information is used for fault diagnosis and detection.The main research contents of this paper include the time domain feature extraction method of bearing vibration signal, frequency domain feature extraction method, and the method of bearing fault diagnosis based on support vector machine .
This paper studies the vibration signal time domain indexes, including the RMS value, variance, peak factor, kurtosis, skewness and sixth order center distance, then the vibration signal of the frequency domain feature extraction method, main peak of bearing fault characteristic frequency is extracted. In the end of this paper, support vector machine is used for bearing fault pattern recognition, and the recognition rate is obtained. The influence of different kernel functions and other parameters on the accuracy of SVM classification is analyzed.
Keywords: rolling bearing;fault diagnosis;support vector machine;feature extraction
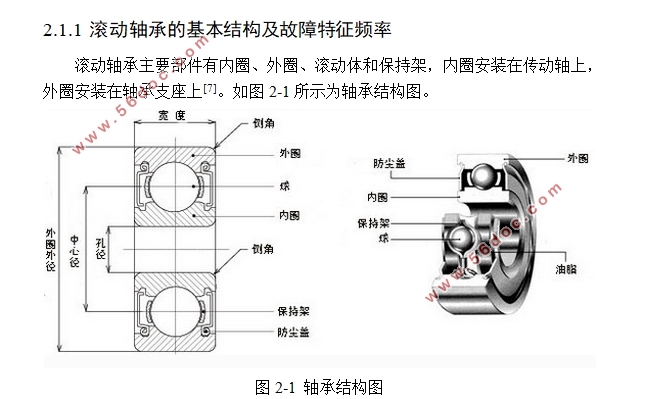
目录
摘要 I
Abstract II
目录 III
1 绪论 1
1.1 研究的目的及意义 1
1.2 滚动轴承故障诊断研究现状 2
1.3 本论文的主要研究内容 3
2 轴承故障诊断与SVM原理 4
2.1 轴承故障诊断原理 4
2.1.1 滚动轴承的基本结构及故障特征频率 4
2.1.2 滚动轴承典型故内圈损伤障的振动特性 5
2.2 SVM原理 7
2.2.1 最优分类面 7
2.2.2 线性不可分的处理原则 8
2.2.3 多分类SVM 11
2.3 小波变换基本原理 12
3 基于SVM的轴承故障诊断 14
3.1 滚动轴承诊断流程 14
3.2 样本数据及特征向量提取 15
3.2.1 轴承试验台及样本数据描述 15
3.2.2 轴承时域特征向量提取 16
3.2.3 轴承频域特征向量提取 19
3.3 SVM分类器训练及测试 20
4 总结与展望 23
4.1 论文工作总结 23
4.2 论文工作展望 23
参考文献 25
致谢 26
|